Context
While overall adoption of AI remains low among businesses, most enterprise executives agree that AI is not just hype. A recent McKinsey Consulting study demonstrates that 40 percent of all the potential value that can be created by analytics today comes from the AI techniques that fall under the umbrella of “deep learning” (which utilize multiple layers of artificial neural networks, so called because their structure and function are loosely inspired by that of the human brain). According to the study, deep learning could account for between $3.5 trillion and $5.8 trillion in annual value.
However, many business leaders are still not exactly sure where they should embed AI to reap the biggest rewards – which functions and use cases. After all, embedding AI across the enterprise requires significant investment in data acquisition, talent and upgrades to the tech stack as well as sweeping “change management” initiatives to ensure AI drives meaningful value.
At BGV we’ve applied the lens of an old adage in answering the question of where to put AI to work: “Follow the money.” The business areas that traditionally provide the most value to companies tend to be the areas where AI can have the biggest impact. Mckinsey has identified that the greatest potential value impact from using AI is both in top-line-oriented functions, such as marketing and sales and bottom-line oriented operational functions, including supply-chain management and manufacturing.
We believe that the functional areas where AI can have the greatest impact are supply-chain management (including manufacturing) as well as sales and marketing. At BGV our own experience with our portfolio companies echoes the sentiments of the McKinsey study. We believe that the most likely functional areas where AI can have an impact are supply chain management (including manufacturing) as well as sales and marketing and underwriting of risk. See McKinsey Consulting chart below:
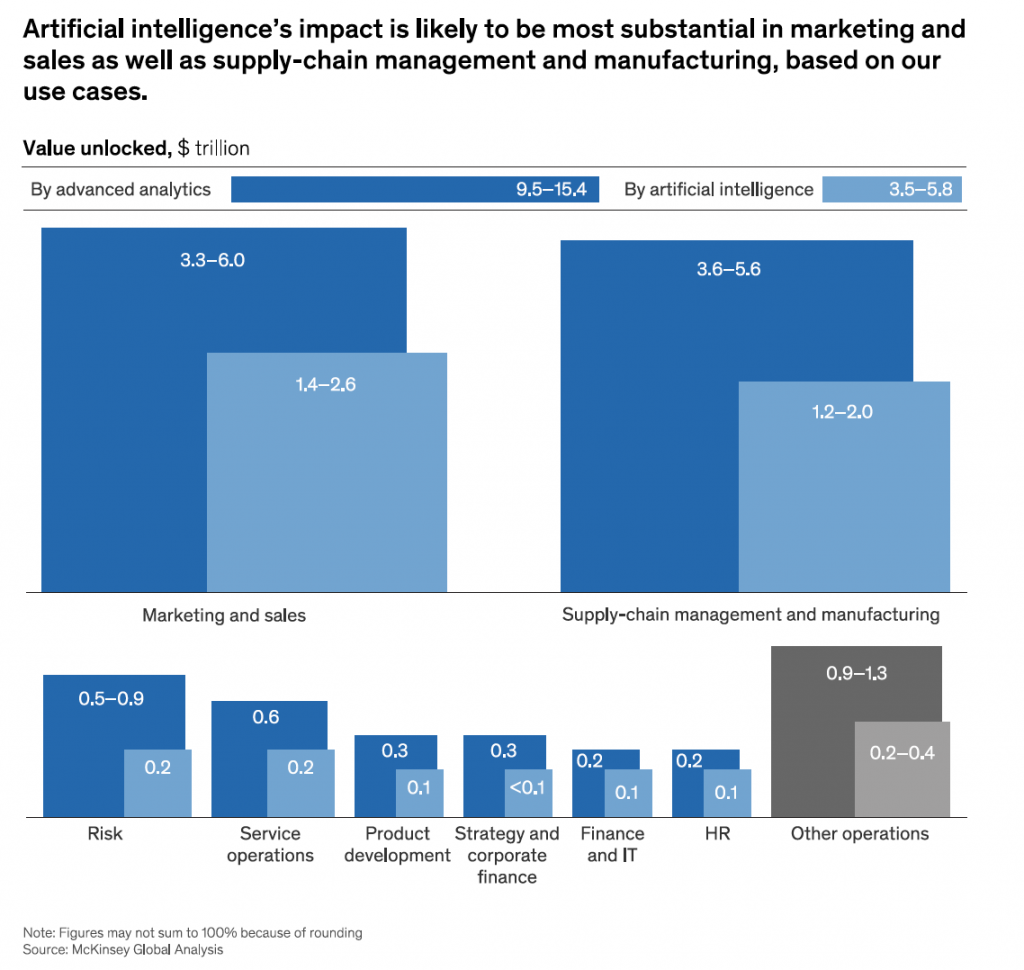
Waves of AI
At BGV, we are convinced that AI has moved beyond the initial wave of research and proof of concepts, progressed through the phase of Cloud AI dominated by CSPs (cloud service providers like Google Cloud and AWS) and is now entering into the phase of the Recommender where it will be fully capitalized by Enterprises (see next chart). A common pattern in each Wave is AI Recommenders and each Wave delivers foundational capabilities to deliver end-to-end Recommendation systems.
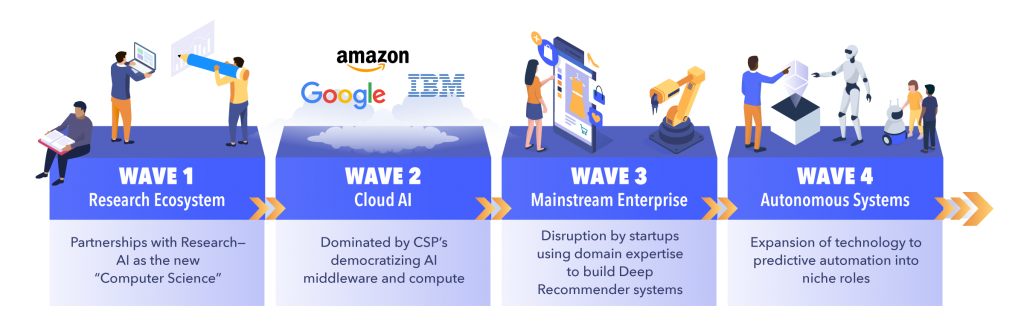
At BGV, we believe that the super use case for AI is deep recommendation systems, ranging from chip development, drug discovery, inventory planning, supply chain management, go-to-market, what products to sell (sales optimization), how to best market (marketing optimization), optimizing customer journeys, automated customer support (customer Service optimization) and core enterprise asset management and utilization. The large CSPs have leveraged their access to massive computing power, data storage and AI algorithm libraries, coupled with sophisticated software development to play a leadership role in democratizing access to AI middleware and compute. In contrast to these, we are convinced that the deep recommender solutions will come from teams with unique solutions and domain knowledge leveraging the capabilities developed by CSPs. These teams will often bring a fresh perspective to a well understood, previously unaddressed business problem. Most AI initiatives in core industries have failed to move beyond Proof of Concept (PoC) because AI systems have traditionally exposed problems but do not necessarily suggest how to solve them. Additionally, AI initiatives have historically vastly underestimated the need to integrate and embed into operations which creates massive friction in adoption. Adoption reinforces and builds trust in the Human-Machine Interface (HMI).
A deep recommender system should systematically address all of these deficiencies – ROI, operational integration and HMI trust. ROI in the first year of deployment is a critical success factor. Often, enterprises will adopt a deep AI recommender system if they result in immediate ROI impact rather than making significant upfront investment for a likely saving in year three or later. ROI spurs deployment which drives more ROI driving positive network effects. Essentially, this will determine the low hanging use cases for AI deep recommender systems. Use cases where spend is happening based on ROI today is around Predictive Analytics solutions (eg C3ai’s success in predictive maintenance for ATMs in financial services and Aircraft maintenance in Aerospace). Furthermore, we believe that AI can replace rules based systems in industries where it can provide superior accuracy and timing. For example providing information two months in advance for specific predictive decisions can create huge value for customers which often is a function of verticalization and adoption.
Our definition of deep recommender AI systems is outlined below:
“AI recommender systems augment and enhance human productivity by extracting signals from Business and Operations data. The AI processing on the data is done in a bias free and explainable way and the AI decisioning is manifested in the natural business workflows used by the human expert. A testament to a good AI recommender system is how inconspicuously and seamlessly it supports the tasks and decision process of the human expert. By virtue of being embedded in the human loop, the recommender is able to learn from human decisions and acquire high fidelity data from human actions, learn to reduce bias, improve explainability and build trust in the overall AI usage with operational decision makers which drives a strong ROI. “
Early Conclusions
BGV Portfolio examples such as Dristhi for Line Manufacturing, Peritus for Technical Support, AiDash for Enterprise Asset Management (EAM) operations and Zelros for Sales & Marketing in Insurtech are exemplars for AI deep recommender systems. While it is still early days, these companies share some important attributes such as strong ROI, access to unique datasets, vertical domain expertise and deep AI technology (encoded domain expertise, coupled with automated learning features). These deep recommender systems will not be standalone or even visible to end users as they strive to make the end user experience seamless. The AI operates with high fidelity and in the background, seamlessly integrating with experts in the decision loop and manifesting value into the same vertical workflows.
We are learning that increasing the scope of deep recommender systems even within a single use case takes time because of trust and learning. Startups must stay focused and walk before they can run. This “land first and then expand” is contrary to the traditional horizontal SaaS growth model of “broad new logo acquisition.” This is essential because of the need to automate the data quality for each “landing.” Without doing so it is difficult to provide good recommendations based on sufficient volume and quality of data. Working on a narrow problem domain/use case helps transfer learning across to each new customer.
Furthermore, companies in this category will need to navigate complex challenges:
- Traceability, which leads to humans trusting the AI models. Making the AI technology evidence based, easily understandable and explainable, particularly in the absence of a clean data set is essential. Decision makers must have clear evidence of how the AI made a decision and the provenance of the steps it took to arrive at that decision. We are finding that coaching and training is an essential part of building trust in AI (more so for blue collar workers than white collar), as is A/B testing to demonstrate that the models are delivering better results than human-only or rules-based methods. This challenge makes it essential for startups to invest sufficiently in UI/UX that optimizes human-machine interactions.
- Ethical use of AI. This top level strategic concern must ensure that algorithms do not introduce gender, racial or other bias into recommendations. The WEF has done solid pioneering work in the area of defining ethical AI principles but startups need to ensure that these principles are translated to the methods and software and that there is sufficient instrumentation and evidence to measure the range of values for ethical outputs.
- Data privacy, Data Provenance and Data Usage. Regulators will look for clear proof that will rate limit adoption, especially in regulated industries like Financial services and Healthcare. There is a need to ensure that companies abide by current and future privacy standards and regulations.
- Human-Machine Trust. AI Recommendation systems built on these core principles can create and unlock tremendous operational value. But the AI decisions have to be trusted. This trust fabric must be integrated into the operational layers of the business system. The Human Trust in AI decisions (what, why and how) accelerates deployment which drives more AI adoption, driving positive network effects and scale ROI.
In conclusion, we believe that the most successful AI startups will be the ones that can make their technology ethical and inclusive, so that it helps the world, not just the bottom line. The winners will be ones that enable better decisions than those made today, based on a system of records and a system of engagements (eg how marketing automation and sales automation businesses have built better value using SFDC’s system of records). This will result in the ability to successfully shift value capture from systems of records and engagement to genuine AI-fueled systems of intelligence.