The technology industry has a well-documented love for creating new buzzwords. Quantum computing, Blockchain, Hyper Automation, Edge Computing, IoT and Connected Retail are prime examples. One can argue that the use of buzzwords is worse in tech sector than in other industries simply because innovative ideas are constantly disrupting the established ecosystems and there is a need to stand out. However, this is a poor excuse for creating a revolving door of terms with murky meanings that create more confusion than clarity.
In this blog Anik Bose and Yash Hemaraj (BGV Partners) and June Zhang (BGV intern and Kellogg MBA candidate) attempt to demystify and share their perspective on the “Edge AI” opportunity.
The Case for Edge AI
Edge AI is defined as the implementation of machine learning algorithms on locally owned computers (or embedded systems) with the need for remote servers. A device using Edge AI does not need to be connected in order to work properly (i.e. can work offline), it can process data and make decisions independently without a data connection. Edge AI only requires that devices possess a microprocessor and sensors.
One may ask, why should AI inference move to the edge? Put simply, that is where the data is. There is little benefit to sending all the data from things like video and audio streaming to cloud servers far away and then back down to the device for every situation. Inference for applications that that require massive computing power (and are not time or latency sensitive) will continue to utilize cloud based AI. However inference for applications that require real time response can enjoy a number of benefits by adopting edge AI such as:
- Real time operations
- Data transport Bandwidth cost savings
- Lower power consumption
- Security and privacy built into the DNA of edge AI
- Scalability
Given the unique requirements for latency, efficiency, privacy, and availability one can expect that edge AI opportunities will inherently differ from those that are cloud based. The edge AI applications will differ based on the device and the vertical market – see data based on BGV internal analysis:
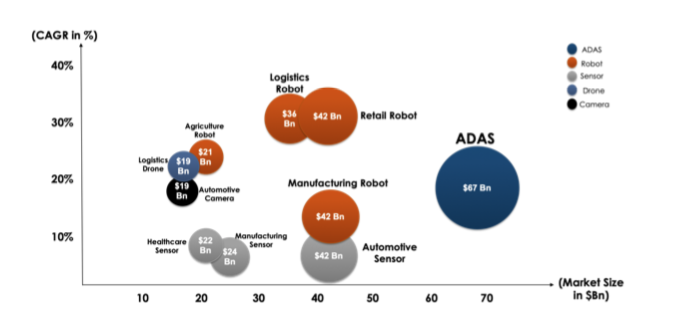
Challenges
While edge AI offers several advantages (enabling real time operations, lower power consumption, scalability, enhanced security, privacy, and costs savings) it also poses several key challenges that may limit it’s adoption. Potential roadblocks include computation capacity, battery life, diversity of application hardware and cost sensitivity (microprocessor and edge device). We believe that three key trends will provide positive tail winds to drive the adoption of edge AI:
- Next Generation Chips: Driven by technologies such as Extreme Ultraviolet Lithography (EUV), a next generation lithography technology that utilizes extreme UV wavelengths to produce more powerful chips at a lower cost. EUV can print transistors using wavelengths of light that are seven times shorter than previous methods. This results in a higher-resolution process that can pack more transistors into the same space. Key applications for this technology involve data intensive tasks (e.g. autonomous driving). Samsung and TSMC are leading EUV chipmakers.
- Federated Learning (FL) – Is a machine learning technique that trains an algorithm using multiple decentralized edge devices or servers, each holding local data samples, without exchanging these samples. This is different from traditional cloud-based machine learning techniques. FL enables multiple actors to build a common, robust machine learning model without sharing data. In this way FL addresses critical issues such as data privacy, data security, data access rights, access to heterogeneous data, and high personalization needs. Key applications are health care, IOT, defense, and telecom. Industry players driving this are Google (Gboard), Apple, NVIDIA, and OpenMind.
- AutoML – Is a suite of AI tools to automate the design and training of neural networks. It will grant broader ML technology by lowering the barrier to entry for enterprises with minimal AI expertise. AutoML helps AI startups and end users skip the time-consuming process of searching for the right neural network architecture and lowers the cost of developing a usable AI tool. It does this all with just small amount of training data. Industry players driving this movement are Google, Databricks, H2O and Data Robots.
Edge AI Investments & Ecosystem
Notable startup activity include:
- Kneron, a San Diego-based startup developing edge computing chips for AI inference. They raised a $40M Series A follow-on round from Horizon Ventures.
- Apple acquired edge AI startup Xnor.ai for $200M.
- Skylo, a California-based startup developing solutions to connect IoT products with satellite networks, raised a $103M Series B funding round led by SoftBank. (IoT infrastructure is attracting more attention as the connectivity tech becomes more common. The satellite IoT market is estimated to be worth $2.86B, according to CB Insights’ Industry Analyst Consensus.)
- Horizon Robotics is a China-based startup developing edge AI chips for autonomous driving, smart city traffic surveillance, and in-store cameras for retail analytics. The company raised a $600M Series B funding led by SK China in February 2019, valuing the company at $3B.
- Falkonry, a California-based AI-IoT startup, launched a “portable, self-contained engine” that can be deployed on edge devices for predictive analysis
Large global vendors in edge AI include:
- Google – Relies heavily on organic growth with a focus on advertising, cloud, ML, and search. Google Coral was officially announced in March 2019. It is a platform of hardware and software components that help build devices with local AI — providing hardware acceleration for neural networks on the edge device.
- Microsoft – Relies on organic growth, such as new product launches and product update. Focus on AI, ML, and IoT technologies. Microsoft offers a comprehensive toolkit called Azure IoT Edge that allows AI workloads to be moved to the edge of the network
- Apple – Relies on inorganic growth. Has purchased more than 20 AI startups since 2010. Focus on AI solutions around Apple smart devices. In Jan 2020, Apple spent $200 million to acquire Xnor.ai, a Seattle-based AI startup focused on low-power machine learning software and hardware
Conclusion
At BGV, our thesis revolves around the emergence of a new class of companies – called Enterprise 4.0 companies – that are transforming industries and combine Intelligent workflows, AI and unique datasets into a complete full-stack solution, monetized with a SaaS business model. In this context, we believe that value creation opportunities for startups in edge AI will be centered around:
- Vertically focused edge device applications. These are Edge AI applications integrated with off the shelf edge devices to deliver a complete “full-stack” solution that we expect to see in Enterprise 4.0 companies. Full stack robotic solutions, for example, are playing a key role in retail, manufacturing and logistics. Similarly, sensor data is powering intelligent workflows that are being used in manufacturing, health care, and the automotive industry.
- While ADAS represents a large potential market opportunity given the oligopolistic nature of the automotive industry and given the capital intensity of such component plays, startups will need large ecosystem partners to deliver complete solutions and the frictionless deployment models required to build venture scale businesses.
- Horizontal opportunities will exist around Auto ML and Federated learning. However, the winning solutions that emerge will tie the “best of breed” capabilities into monetizable solutions that tie usage to value capture opportunities.
BGV has seen several cycles of innovation work through the enterprise technology landscape, and our partners have stood at the forefront of these movements. This fourth wave, Enterprise 4.0 has now emerged, and we are excited to work with game changing disruptors in this space. If you are an entrepreneur building an edge AI company along these lines, then we would welcome the opportunity to collaborate with you.